Recognizing Handwritten Digits with Scikit-Learn
Recognizing handwritten text is a problem that traces back to the first automatic machines that needed to recognize individual characters in handwritten documents. Think about, for example, the ZIP codes on letters at the post office and the automation needed to recognize these five digits. Perfect recognition of these codes is necessary to sort mail automatically and efficiently. Included among the other applications that may come to mind is OCR (Optical Character Recognition) software. OCR software must read handwritten text, or pages of printed books, for general electronic documents in which each character is well defined. But the problem of handwriting recognition goes farther back in time, more precisely to the early 20th Century (the 1920s), when Emanuel Goldberg (1881–1970) began his studies regarding this issue and suggested that a statistical approach would be an optimal choice.
To address this issue in Python, the scikit-learn library provides a good example to better understand this technique, the issues involved, and the possibility of making predictions.
scikit-learn library:
The scikit-learn library (http://scikit-learn.org/) enables us to approach this type of data analysis in a way that differs slightly from what we’ve used in the previous project. I closely related the data to be analyzed to numerical values or strings, but can also involve images and sounds.
Aim:
The primary aim of this project involves predicting a numeric value, and then reading and interpreting an image that uses a handwritten font.
we will have an estimator with the task of learning through a fit() function, and once it has reached a degree of predictive capability (a model sufficiently valid), it will produce a prediction with the predict() function. Then we will discuss the training set and validation set created this time from a series of images.
The hypothesis to be tested:
The Digits data set of the scikit-learn library provides numerous datasets that are useful for testing many problems of data analysis and prediction of the results. Some Scientist claims that it predicts the digit accurately 95% of the times. Perform data Analysis to accept or reject this Hypothesis.
The Digits Dataset
The scikit-learn library provides many datasets that are useful for testing many problems of data analysis and prediction of the results. Also in this case there is a dataset of images called Digits. This dataset comprises 1,797 images that are 8x8 pixels in size. Each image is a handwritten digit in grayscale.
Implementation :
Import libraries
this project uses python libraries numpy, sklearn, matplotlib.
Load digit dataset as dig_data
Scikit-learn provides numerous datasets. And the digits dataset is one of those, which contains set of around 1800 images each sizes 8x8 pixels in size. We get more information related to our dataset by using DESCR method of dataset.
Lets get to know more about the dataset dig_data and what it consists of. This dataset is Bunch type object. Bunch object is similar to a dictionary with multiple values for a specific key. The important keys of dig_data are images(bits of image data), target(the true value of image), target_names(different allowed outputs).
Seperate the dig_data.images and assign it to digits variable. The digits is reshaped into a 2-dim array.
Now the input to the classifier model digits(dig_data.images) as X and output dig_data.target as Y are split into two parts the train_data and test_data in the ratio 7:3 using sklearn.model_selection.train_test_split.
Define the SVC classifier as model and train model using model.fit() method with X_train, Y_train as inputs. After training the model, check the score of the model and accuracy_score from sklearn.metrics.
To evaluate the accuracy of a classification model a confusion matrix is used. A confusion matrix is a table that shows the prediction results in X-axis with respect to true values in Y-axis. The below plot is constructed using plot_confusion_matrix function in sklearn.metrics.
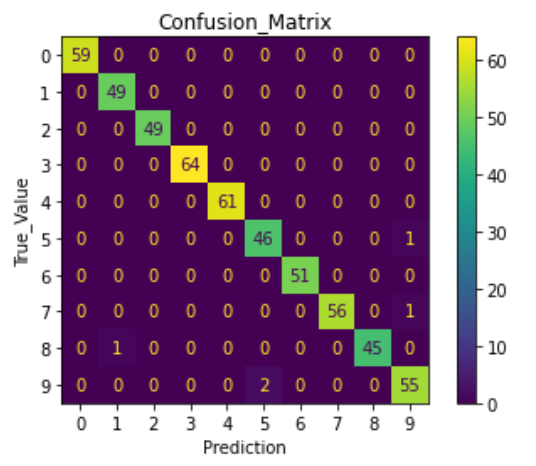
Conclusion
After performing the data analysis on the dataset with three different test cases, we can conclude that the given hypothesis is true i.e., the model predicts the digit 95% accurately.
Source code: https://github.com/mukunddholariya/suvenconsultants/blob/main/Recognizing%20Handwritten%20Digits%20with%20scikit-learn/Recognizing_Handwritten_Digits_with_Scikit_Learn.ipynb
I am thankful to mentors at https://internship.suvenconsultants.com for providing awesome problem statements and giving many of us a Coding Internship Experience. Thank you www.suvenconsultants.com
Comments
Post a Comment